-
Financial administration
An accurate financial administration provides you with the information you need to take the right decisions. The big advantage of a digital financial administration is that it provides insight into your most important financial processes at any time, whether this is the invoices, salary payments or bank changes.
-
Financial insight
You want to take the right decisions, based on trustworthy and clear management information. You want to have access to all your financial data, 24/7, in order to determine your position and be able to adjust where necessary.
-
Global compliance partnering
Outsourced compliance services comprises the total financial compliance of your business, in accounting, financial reporting, payroll, legal and various tax reporting obligations. We can make sure you don’t have to worry.
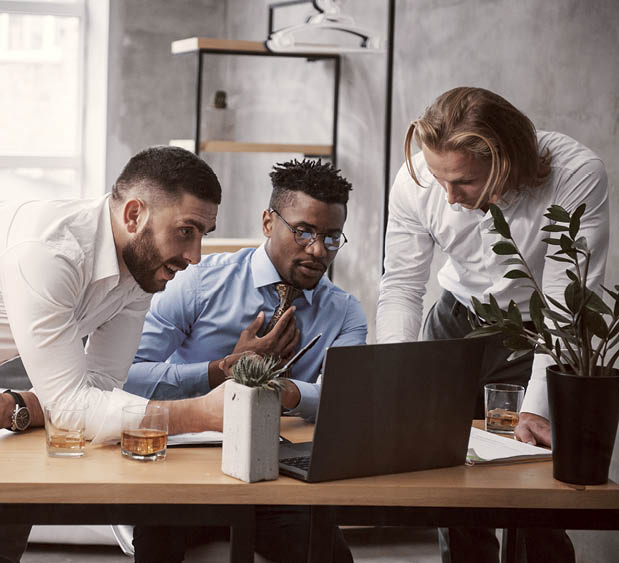
-
Impact House by Grant Thornton
Building sustainability and social impact. That sounds good. But how do you go about it in the complex world of stakeholders, regulations and frameworks and changing demands from clients and society? How do you deal with important issues such as climate change and biodiversity loss?
-
Business risk services
Minimize risk, maximize predictability, and execution Good insights help you look further ahead and adapt faster. Whether you require outsourced or co-procured internal audit services and expertise to address a specific technology, cyber or regulatory challenge, we provide a turnkey and reliable solution.
-
Cyber risk services
What should I be doing first if my data has been kidnapped? Have I taken the right precautions for protecting my data or am I putting too much effort into just one of the risks? And how do I quickly detect intruders on my network? Good questions! We help you to answer these questions.
-
Deal advisory
What will the net proceeds be after the sale? How do I optimise the selling price of my business or the price of one of my business activities?
-
Forensic & integrity services
Do you require a fact finding investigation to help assess irregularities? Is it necessary to ascertain facts for litigation purposes?
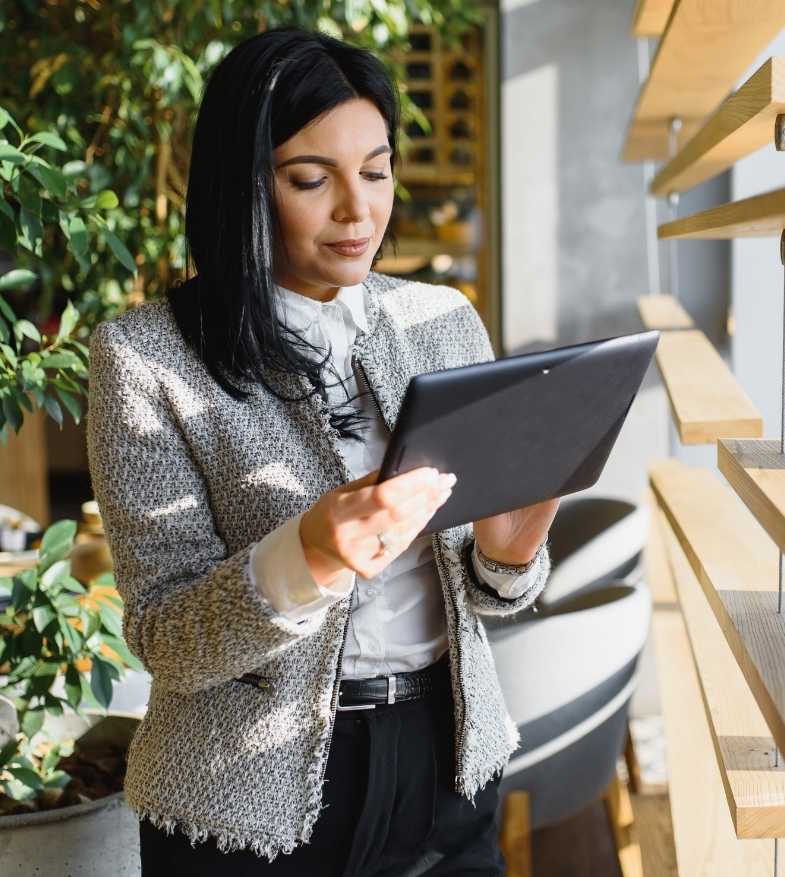
-
Auditing of annual accounts
You are answerable to others, such as shareholders and other stakeholders, with regard to your financial affairs. Financial information must therefore be reliable. What is more, you want to know how far you are progressing towards achieving your goals and what risks may apply.
-
IFRS services
Financial reporting in accordance with IFRS is a complex matter. Nowadays, an increasing number of international companies are becoming aware of the rules. But how do you apply them in practice?
-
ISAE & SOC Reporting
Our ISAE & SOC Reporting services provide independent and objective reports on the design, implementation and operational effectiveness of controls at service organizations.
-
Pre-audit services
Pre-audit services is all about making the company’s entire financial administration ready for checking before the external accountant begins his/her audit of the annual accounts.
-
SOx law implementation
The SOx legislation dictates that management is structurally accountable for reporting on the internal control relevant to the financial statements.
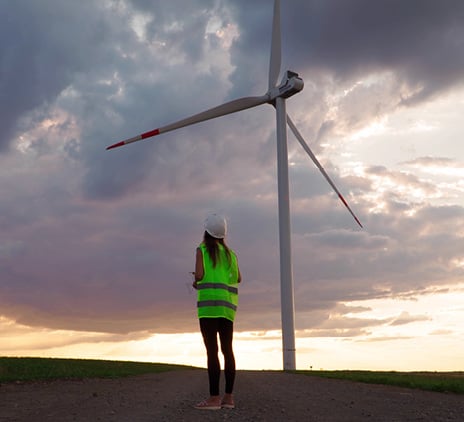
-
International corporate tax
The Netherlands’ tax regime is highly dynamic. Rules and the administrative courts raise new challenges in fiscal considerations on a nearly daily basis, both nationally and internationally.
-
VAT advice
VAT is an exceptionally thorny issue, especially in major national and international activities. Filing cross-border returns, registering or making payments requires specialised knowledge. It is crucial to keep that knowledge up-to-date in order to respond to the dynamics of national and international legislation and regulation.
-
Customs
Importing/exporting goods to or from the European Union involves navigating complicated customs formalities. Failure to comply with these requirements usually results in delays. In addition, an excessively high rate of taxation or customs valuation for imports can cost you money.
-
Human Capital Services
Do your employees determine the success and growth of your organisation? And are you in need of specialists which you can ask your Human Resources (HR) related questions? Human Resources (HR) related questions? Our HR specialists will assist you in the areas of personnel and payroll administration, labour law and taxation relating to your personnel. We provide you with high-quality personnel and payroll administration, good HR guidance and the right (international) advice as standard. All this, of course, with a focus on the human dimension.
-
Innovation & grants
Anyone who runs their own business sets themselves apart from the rest. Anyone who dares stick their neck out distinguishes themselves even more. That can be rather lucrative.
-
Tax technology
Driven by tax technology, we help you with your (most important) tax risks. Identify and manage your risks and become in control!
-
Transfer pricing
The increased attention for transfer pricing places greater demands on the internal organisation and on reporting.
-
Sustainable tax
In this rapidly changing world, it is increasingly important to consider environmental impact (in accordance with ESG), instead of limiting considerations to financial incentives. Multinational companies should review and potentially reconsider their tax strategy due to the constantly evolving social standards
-
Pillar Two
On 1 January 2024 the European Union will introduce a new tax law named “Pillar Two”. These new regulations will be applicable to groups with a turnover of more than EUR 750 million.
-
Cryptocurrency and digital assets
In the past decade, the utilization of blockchain and its adoption of a distributed ledger have proven their capacity to revolutionize the financial sector, inspiring numerous initiatives from businesses and entrepreneurs.
-
Streamlined Global Compliance
Large corporations with a presence in multiple jurisdictions face a number of compliance challenges. Not least of these are the varied and complex reporting and compliance requirements imposed by different countries. To overcome these challenges, Grant Thornton provides a solution to streamline the global compliance process by centralizing the delivery approach.
-
Expand into new markets
Do you seek for opportunities in the global business arena? Whether you are about to open a new office in a foreign country or considering an international acquisition, you need certainty of making the right choices for your company. Global expansion isn’t always as simple as it sounds. The good thing is that we’re here to help!
-
Expanding your business in the Netherlands
International expansion is an important step. The Netherlands can be your gateway to Europe for doing business abroad. But why you should choose the Netherlands?
-
Global contacts
Wherever you choose to do business, you want access to people with the best ideas and critical thinking that will enable you to grow your business at home and abroad.
-
Corporate Law
From the general terms and conditions to the legal strategy, these matters need to be watertight. This provides assurance, and therefore peace of mind and room for growth. We will be pro-active and pragmatic in thinking along with you. We always like to look ahead and go the extra mile.
-
Employment Law
Small company or large multinational: in any company your people are of the utmost importance for your business. Employment brings with it many issues in many areas and often has legal consequences. For big strategic, but also for more everyday questions about employment law, our lawyers are ready to help you out. Also for questions about international employment law. Do you have your own HR department? We’ll gladly assist them. We deliver bespoke services and are there when you need us.
-
Sustainable legal
Sustainability is more than a buzzword - it is the core of our legal advice towards sustainable success. From drafting sustainable contracts, integrating sustainable HR policies and ESG due diligence within our M&A practice to advising on ESG and other (national and international) legislation: we prefer to be pragmatic and proactive in helping your business.
-
Maritime sector
How can you continue to be a global leader? The Netherlands depends on innovation. It is our high-quality knowledge which leads the maritime sector to be of world class.
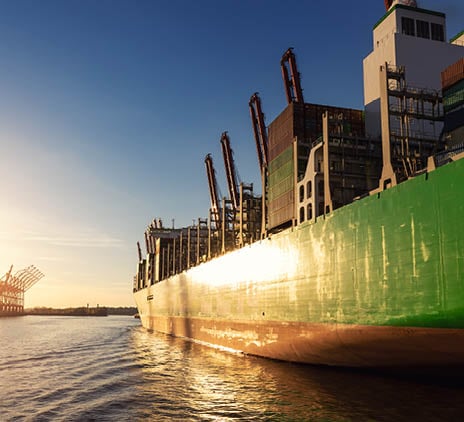
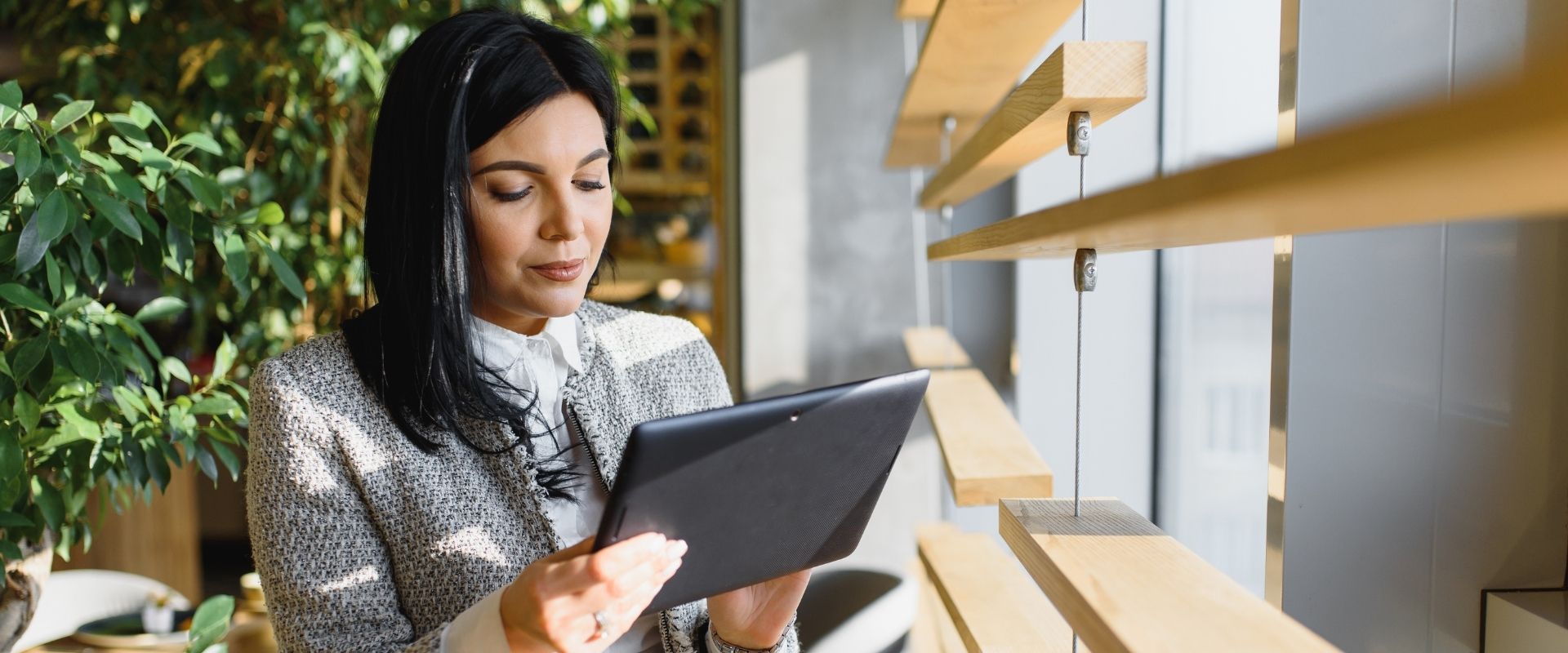
What is Data Quality Assurance (DQA)?
Data Quality Assurance (DQA) ensures data is in the right format and structure without errors before and during the acquisition to serve your strategic company goals and purposes. It detects inconsistencies and anomalies by performing data cleansing, eliminating obsolete information, removing outliers, and fulfilling missing data at an earlier stage.
Why data quality assurance?
As data specialists, we spend a large amount of time preparing data for analytics. From our experience, we know that data quality is one of the biggest struggles and challenges you can face when analyzing data. We know the importance and the role of data quality in performing accurate analysis and generating good reports to help you make optimal decisions.
The importance of data quality assurance:
Risk Mitigation
Organisations nowadays collect huge amounts of data. This data becomes an important asset for organisations, and just like any other asset, it has some value and therefore has some level of risk of getting modified or changed by internal and external factors and individuals. Influencing the state of data quality and therefore increasing the risk of compliance issues due to inaccurate reporting, can lead to fines and regulatory scrutiny.
Implementing data quality assurance procedures will help you to identify and address data issues that could lead to business risks and therefore take the right action at the right time. This way you can reduce risks related to data errors that affect your business’ operations and results.
Improving data quality reduces the risk of penalties and enhances regulatory compliance by ensuring and simplifying accurate compliance reporting and audits. Moreover, it helps your organisation to minimize risks associated with non-compliance, such as fines and reputational damage.
Data quality controls
Data Quality Assurance can help your organisation to protect the value of your data and define SMART metrics and measures that can help implement effective data controls to manage data quality audits more efficiently. Data Quality Assurance can also help implement policies, strategies, and governance measures to remediate different data security issues.
Decision-making improvement
Making the right decision requires high-quality data that helps generate good reports and get accurate and reliable insights therefore enabling better business decisions.
As data specialists, we use analytics daily to generate reports that help our financial audit colleagues with better and faster data analysis for the audit. However, sometimes due to the inaccurate data from the client’s systems, it becomes a challenge to get optimal results to help managers make better audit conclusions.
By establishing data quality procedures in our clients' organisations, these organisations can improve data quality management and therefore help generate data quality rules compatible with business data governance which will ensure the accuracy of data used for analysis and decision-making.
Cost Savings
Improving data quality may help reduce the time and resources needed to prepare data for the analysis, it can help reduce the inefficiencies associated with poor data quality therefore leading to cost reductions by reducing the amount of time spent on cleansing and correcting data errors.
Examples from our daily work show how bad-quality data can affect data analytics tasks and make it expensive for clients who struggle with inconsistent sales and purchase data which is entered manually. Entering data manually leads to inaccurate data which makes data analytics impossible or at a very high cost with the risk of wrong results.
AI and Competitive Advantage
Bad data quality may be a barrier to the use of data analytics and AI/machine learning models to gain competitive advantages. Competitive advantage based on modern technologies comes from creating business models that use AI and digital innovation where data quality is the heart of implementing these modern information technologies.
Implementing data quality assurance will help businesses differentiate from their competitors and allow organisations to bring their objectives to the next level by enabling them to use AI and machine learning models and techniques to achieve more accurate, efficient, and scalable solutions and therefore gain competitive advantages.
Moreover, improving data quality can support companies to develop new products and services, as a result, it can improve their market positioning and strategic decisions.
More information on data quality assurance?
If you want to know more about the importance of data quality assurance for your organisation, please get in touch with our Business Risk Services team to see how Grant Thornton can offer bespoke support for your data quality projects.